
DEI/CISUC Seminars
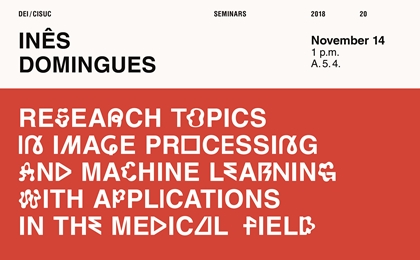
November 14, Wednesday,
13h (sharp),
Room A.5.4. DEI-FCTUC
Invited Speaker: Inês Domingues
Title: "Research topics in image processing and machine learning with applications in the medical field"
Abstract: In this talk I will provide an overview of my post-doctoral research. I develop image processing and machine learning techniques for medical contexts. I have been particularly interested in three main lines of research: scarcity of labelled data, imbalance, and interpretability. Deep learning has raised a lot of interest lately due to its increased performance when compared with non-deep techniques. However, deep learning methods require a large amount of data to train on. When focusing on supervised learning, the training data needs to be labeled. In medical contexts, labeled data is very expensive to obtain. We have therefore proposed a data augmentation technique that takes into consideration the anatomic soundness of the images.
Another characteristic frequently present in medical data is label imbalance. For example, most of the population is healthy with only a few subjects with a given pathology. While binary labelling can be informative, medical data is often classified into ordinal scales, accordingly to the degree of severeness of the disease, for example. Oversampling is frequently used to balance data in binary and even multi-class datasets. However, the extension to ordinal data has received much less attention. To this end, we have assessed a set of oversampling techniques applied to ordinal datasets, and we are developing oversampling methods tailored to the ordinal case.
In order to deploy the deep learning models in the real world medical contexts, it is highly advisable that they are interpretable in order to increase the trust of the user on the model. We have applied mimic learning to our ordinal settings, making the necessary adjustments. Mimic learning, has a teacher model (typically highly complex) and a learner model (simpler), that profits from the information present in the teacher model.
As future work, we plan to continue developing this research in applications such as 3D segmentation of tumours and anatomic regions, as well as breast cancer detection.
Short bio: Inês Domingues graduated in Applied Mathematics in the School of Sciences at the University of Porto in 2004, completed the Master in Electrical and Telecommunications Engineering at Aveiro University in 2008 and finished the PhD in Electrical and Computer Engineering in the School of Engineering at the University of Porto in 2015 (Cum Laude). She is currently a post doctoral researcher in the ESTIMA project (IPO Porto + Universidade de Coimbra) and an Invited Assistant Professor at DEIS-ISEC.
<!--[if gte mso 9]><xml>